ChatGPT Faces Competition as Bots Navigate Copyrights
Meanwhile, high-quality data is costly and getting scarce, which impedes advances in generative AI
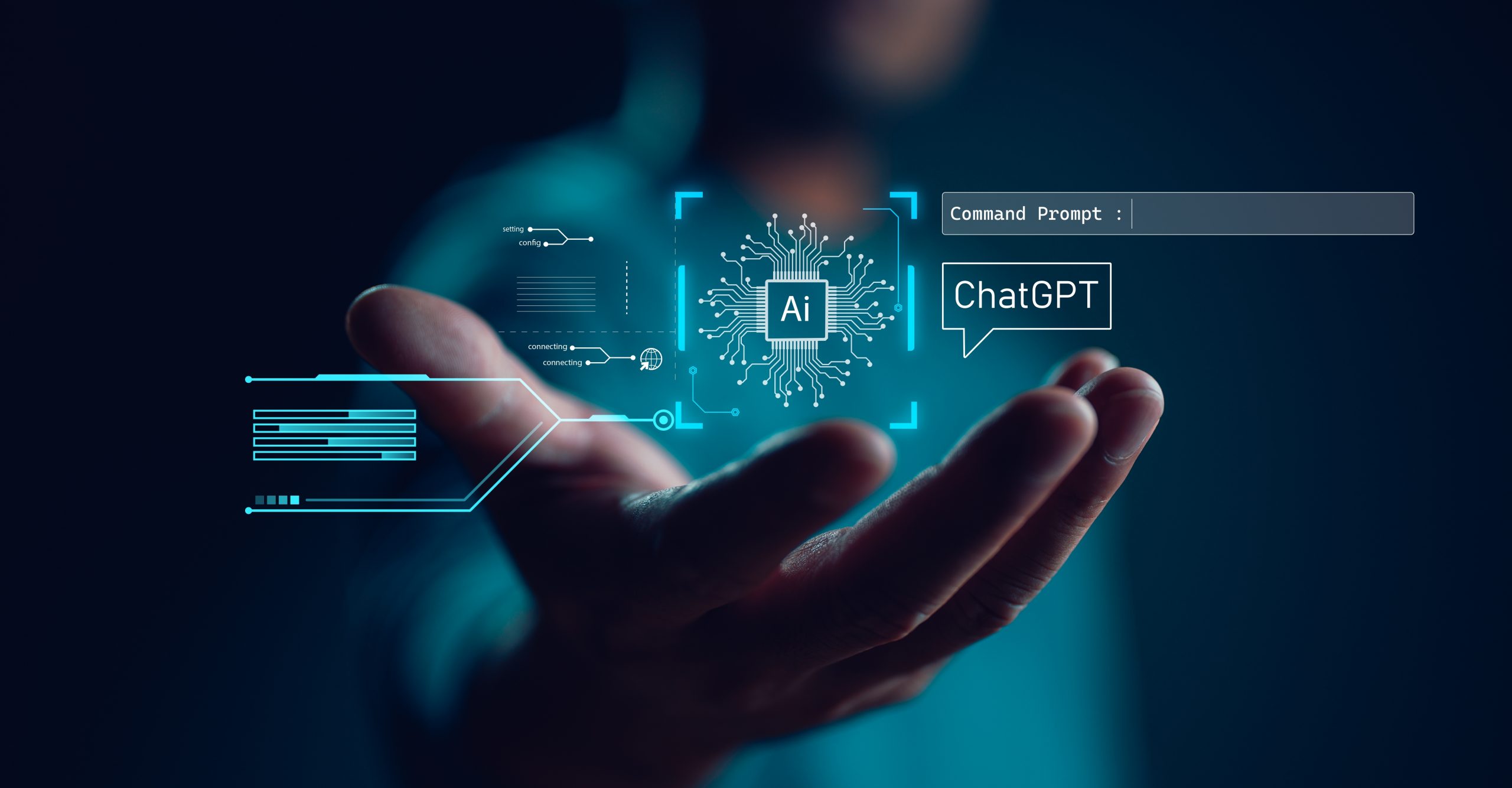
- Consumer adoption of the ChatGPT generative AI platform has slowed, with the number of average monthly users now hovering around 180 million.
- Competition from other generative AI platforms, such as Alphabet’s Gemini and Anthropic’s Claude, has undoubtedly contributed to slowing adoption of ChatGPT.
- However, the scarcity and costliness of high-quality data also represent serious challenges for the industry, and could impede the next big leap in generative AI.
ChatGPT became an immediate global sensation upon its introduction in November 2022, attracting 100 million active monthly users in just two months. By the end of March 2024, that number had swollen to 180 million.
Monthly web visits to ChatGPT grew just as dramatically, soon leaping from zero to 1.5 billion. But they appear to have peaked in May 2023 at about 1.8 billion and then fell as low as 1.5 billion late last year, according to Similarweb. (See illustration below.)
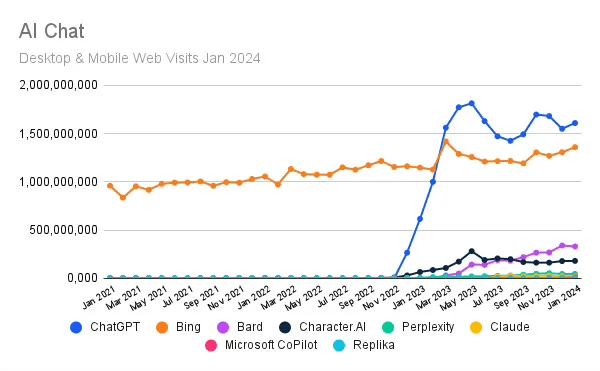
Competition leads the list of possible reasons for consumers’ waning interest in ChatGPT. It ate away at the first-mover advantage. Alphabet (GOOGL), for example, began offering Bard—which was renamed Gemini in February. Its monthly web visits have risen steadily since early last spring, as highlighted in the above chart.
Gemini’s capabilities are on par with those of ChatGPT4, performance data indicates. That probably helps explain—at least in part—why user interest in ChatGPT has slowed.
Besides, Gemini produces AI-powered answers to queries directly below the Google search box (aka “Google search bar”), undoubtedly helping Alphabet usurp some of ChatGPT’s market share.
But the competition doesn’t stop there. Microsoft (MSFT) has Bing, Anthropic offers Claude 2, Adobe (ADBE) sells Sensei and Github markets Copilot. All have been nipping at ChatGPT’s heels.
Looking beyond the competitive landscape, another serious impediment to adoption of generative AI relates to the quality of the platforms’ responses. In January, a research paper published by arXiv indicated ChatGPT-3 “agreed with incorrect statements between 4.8 % and 26% of the time, depending on the statement category.” So, a lack of high-quality data appears to be another problem.
High-quality data is essential for generative AI because it serves as the foundation for training, enabling chatbots to learn the necessary patterns, relationships and structures required to render accurate, comprehensive and unbiased responses. Unfortunately, procuring, organizing, storing and processing high-quality data can be costly.
As a result, today’s most advanced AI platforms are arguably trained on suboptimal datasets, which is why they frequently produce errors, biases or incomplete responses. According to James Vincent, a writer for The Verge, a tech website, “This means they have a tendency to present false information as truth since whether a given sentence sounds plausible does not guarantee its factuality,” he wrote.
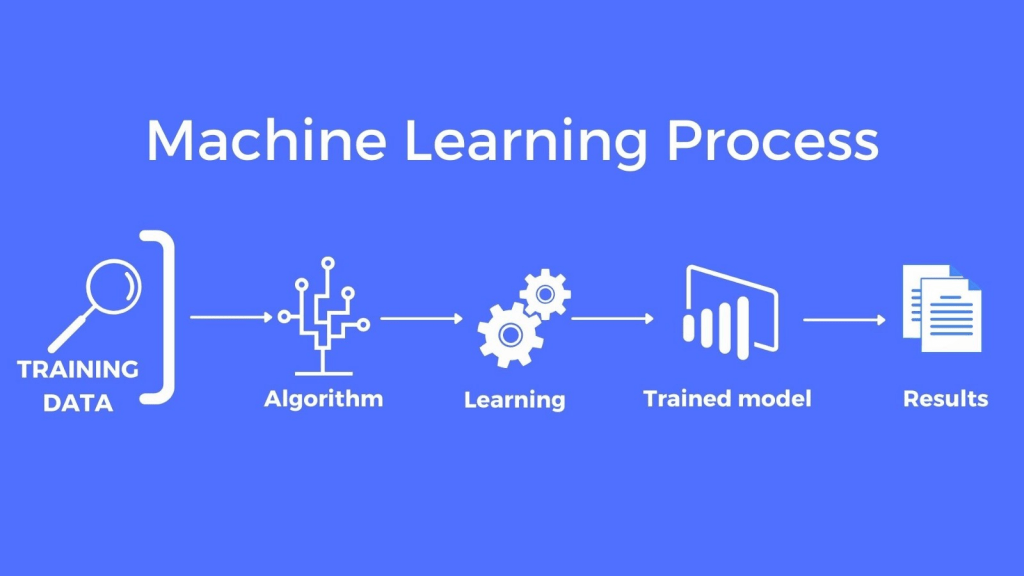
The data conundrum in generative AI
The high-quality date AI needs for training can be prohibitively expensive. For example, Lambda estimated ChatGPT-3 probably cost around $4.6 million to train.
But the training cost for ChatGPT-4 ballooned exponentially higher because it encompassed a much vaster pool of data. It reportedly required roughly 12 trillion tokens (unique data bits that are fed into the model), ChatGPT-4 cost closer to $100 million to train. And with ChatGPT-5 expected to require 60 trillion to 100 trillion tokens, the cost of training could exceed $500 million and might climb as high as $800 million.
Those astronomical figures show why pundits liken data to gold in the new world of generative AI. And according to a recent report by The New York Times, some companies have been forced to “cut corners” to secure the data necessary to train their models.
For example, OpenAI—the privately held company that created ChatGPT—reportedly bolstered its training data reserves by creating a speech recognition tool called Whisper. This tool was then used to “transcribe the audio from YouTube videos, yielding new conversational text that would make an A.I. system smarter.”
Note that The New York Times is suing OpenAI and its partner Microsoft for allegedly “infringing on its copyrights by using millions of its articles to train A.I. technologies like the online chatbot ChatGPT.”
Circling back to the procurement of training data, other creators of generative AI platforms have used similar approaches. According to the report by The New York Times, Alphabet (GOOGL) also used YouTube content to help train its AI models.
Moreover, Google’s need to beef up its available training data is believed to be a key reason the company elected to change its “terms of service” back in 2023. This modification allowed the company to mine data from public Google Docs, restaurant reviews and other online material.
The data at Common Crawl has also been an essential building block for many generative AI platforms, according to CMSWire. Common Crawl’s data set is more than 9.5 petabytes in size and therefore represents “the largest freely available source of web crawl data.” A study by the Mozilla Foundation revealed 64% of the 47 large language models it examined were trained using Common Crawl data.
Creators of generative AI models will devise new, innovative methods to identify and procure the data required to train their models. For example, Alphabet recently announced a licensing agreement with Reddit (RDDT) that enables it to use the social media company’s internal data warehouse of user posts and comments.
However, with the high cost (and growing scarcity) of high-quality data, AI models may need to be trained on what’s known as “synthetic data.” It’s generated by computers instead of human sources. The output from generative AI models is one example. The risk is this approach could weaken the diversity of the training data and contribute to bias and inaccuracy.
Speaking of the future, OpenAI CEO Sam Altman recently said this: “As long as you can get over the synthetic data event horizon, where the model is smart enough to make good synthetic data, everything will be fine.” However, the fact that a prestigious machine learning conference recently banned authors from using generative AI to write scientific papers, suggests the event horizon hasn’t arrived.
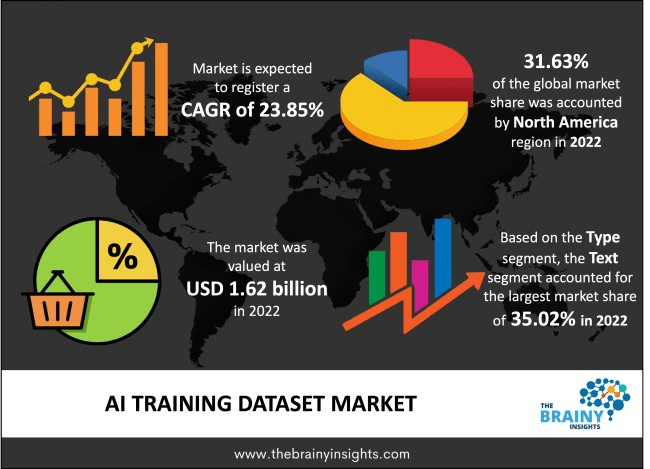
The complication of copyrights—aggrieved authors, artists and musicians
Ultimately, the difficulty and cost of accessing large, diverse data pools helps underscore why generative AI could be dominated by companies with the deepest pockets. Even then, the cost of the most valuable data is so high that it’s not economically feasible for any single entity to pay the toll to legally access it.
Speaking to this complication, Sy Damle, a lawyer for Andreessen Horowitz, told The New York Times that “the only practical way for these tools to exist is if they can be trained on massive amounts of data without having to license that data. The data needed is so massive that even collective licensing really can’t work.”
Obviously, the creators of proprietary works—such as books, articles, music and art—have their own view on the AI training process. Most artists, authors and musicians don’t want to give their work away for free and without their permission. As noted previously, The New York Times has already sued OpenAI and Microsoft for infringing on its copyrights.
For its part, the AI sector justifies its use of copyrighted materials through the “fair use” doctrine. Fair use is a legal principle that allows for the limited use of copyrighted material without the need for permission from (or payment to) the copyright holder. Examples include criticism, commentary, news reporting, teaching, scholarship and research.
When rendering judgements on fair use, courts have historically weighted factors such as the purpose/character of the use, the nature of the copyrighted work, the amount or substantiality of the portion used, and the effect of the use on the potential market for (or value of) the copyrighted work.
Owners of generative AI models have argued that the fair use doctrine applies to their situation because they “transform” the original content for a different purpose and use only a portion of the work, not the whole thing. Moreover, the AI sector argues their use of the copyrighted content doesn’t impact the end market for the original.
New federal and state laws, as well as corporate governance rules, will undoubtedly be unveiled to help clarify how copyright laws will be managed and enforced in the emerging AI era. Along those lines, the U.S. Copyright Office is currently updating its guidance on the topic and is expected to weigh in soon.
It’s unlikely, however, that the forthcoming policy guidance will resolve this complex issue. Instead, it will likely be clarified through a combination of government guidance, legislative action, judicial decisions and established precedents.
In the meantime, the generative AI sector may not be able to advance as quickly as hoped, at least until it finds a way to access deeper pools of high-quality data at a cost that’s economically feasible. That means the next major wave of consumer adoption may be delayed, as potential users hold out for a higher quality products and service.
These issues also help explain why the initial public offering (IPO) of Databricks is so highly anticipated on Wall Street.
Andrew Prochnow has more than 15 years of experience trading the global financial markets, including 10 years as a professional options trader. Andrew is a frequent contributor Luckbox magazine.
For live daily programming, market news and commentary, visit tastylive or the YouTube channels tastylive (for options traders), and tastyliveTrending for stocks, futures, forex & macro.
Trade with a better broker, open a tastytrade account today. tastylive, Inc. and tastytrade, Inc. are separate but affiliated companies.