The Prediction Trade
Science with a touch of art has replaced mysticism as the preferred method of foretelling what lies ahead
The word “Superforecasting” doesn’t carry any hidden meaning. “Super” means far above average, and “forecasting” is the act of making predictions. So, Superforecasters are simply much better than most people at calculating what lies ahead, whether the subject is stocks, options, elections, sports, warfare or just about anything else.
Search “Superforecasting,” and most of the results point to a book that came out five years ago: Superforecasting: The Art and Science of Prediction by Philip E. Tetlock, a professor at the University of Pennsylvania and its Wharton Business School, and Dan Gardner, a senior fellow at the University of Ottawa’s Graduate School of Public and International Affairs.
In their book, Tetlock and Gardner contend that most “experts” make predictions with accuracy that’s only slightly better than chance. But with training, clear thinking and scientific rigor—as well as a measure of natural talent and some good mental habits—Superforecasters learn to make better-than-average prognostications and can even beat the subject-matter experts by a wide margin.
The book springs from the work Tetlock pursued with Barbara Mellers, his spouse and research partner. The couple led a University of Pennsylvania team called the Good Judgment Project that identified and trained Superforecasters as part of a government-led tournament of forecasting (see “The Superforecasters,” p. 22).
Tetlock and Mellers later started Good Judgment Inc., a commercial enterprise that uses Superforecasters to make forecasts and trains forecasters for businesses and governmental agencies (see “Forecasting as a Livelihood,”below).
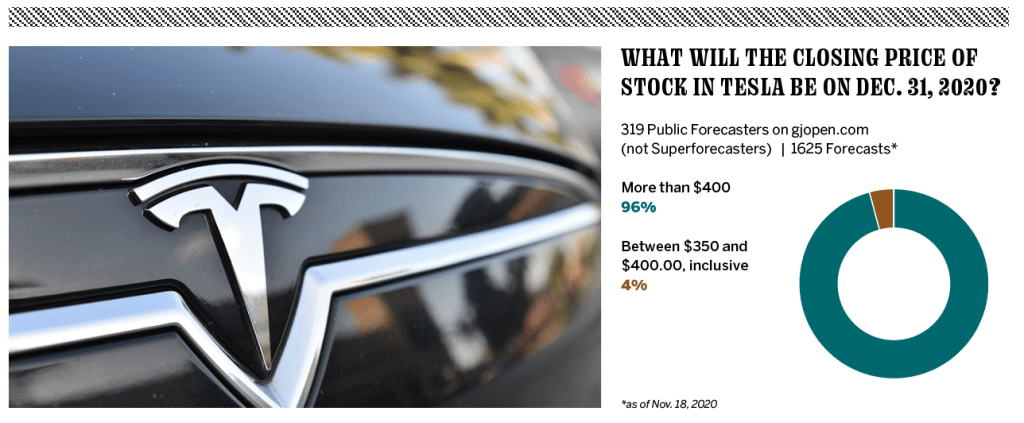
Art or science?
Outsiders often wonder if Superforecasters, who are formally defined as the top 2% of forecasters, approach prediction as an art or science. The Good Judgment Project researchers answer that the ideal forecasting model employs both but leans a bit more toward science.
It begins with science because Superforecasters take the “outside view,” as described in the work of Israeli scientist and Nobel laureate Daniel Kahneman. That means starting the forecasting process with data and establishing a base rate.
But the work doesn’t end with the data—Superforecasters don’t just crunch numbers. The art comes in when they evaluate other factors relevant to the situation.
Take the example of predicting the outcome of an election. Researchers can quantify the power of incumbency based on history. That’s the outside view. But they shouldn’t discount the influence of a scandal. It’s the inside view.
Balancing the inside and outside views to predict the result requires the deft touch of an artist.
But Superforecasters shouldn’t work alone on the tasks of quantifying and then qualifying information. The best predictions evolve from the interplay of Superforecasters.
When Superforecasters with diverse backgrounds arrive at the same conclusion by following different paths, their combined knowledge yields an extremely strong conclusion. If one arrives at Conclusion X via Path A, and the other reaches Conclusion X by traveling Path B, their combined confidence in Conclusion X increases. Stated another way, if both say Candidate A has an 80% chance of victory, combining their forecasts might yield a 90% chance of victory for Candidate A.
But that’s not the only good way of predicting future events based on the wisdom of the crowd.
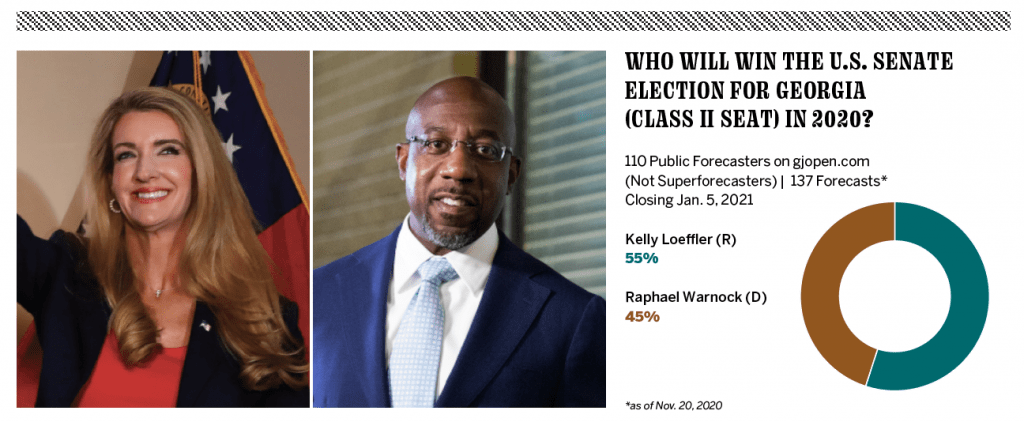
Polls and prediction markets
The Superforecasting experts respect polls and prediction markets but emphasize that the two models are based on different questions. Pollsters might ask who will get a respondent’s vote. Prediction markets ask who a bettor thinks will win the election.
In other words, the poll asks what the respondent is going to do, and the prediction market is based on what betters think will happen.
If a poll of 100 people shows a 55 to 45 split, that means one candidate has a 90% probability of winning because whoever’s leading by 10 percentage points wins 90% of the time. So, a candidate can have a high probability of winning even if the margin’s thin.
Still, forecasting is trickier than that statement might suggest. It’s difficult to keep irrelevant factors out of the mix.
Noise
Bias almost inevitably creeps into the forecasting process. Once it’s identified, forecasters can work to counteract it, but identifying it is the hard part. In fact, some researchers find that bias is hardwired into the human psyche and nearly impossible to eradicate.
One way of detecting bias is to recognize that a forecaster is repeating a viewpoint, data set or piece of information that doesn’t correlate with what really happens. An economist, for example, might keep predicting year after year that a double-dip recession appears imminent, but one almost never occurs. In another example, nearly every press release on almost any corporate merger cites important synergies, but many of the combined companies don’t work out as planned.
Thus, the economist’s predictions and the corporate executives’ rationales amount to nothing but noise. Retrospect reveals such noise, but recognizing it in advance remains a formidable task.
Take the example of the recent presidential election. President Trump’s polling numbers seemed impervious to criticism and allegations of scandal. If pundits had kept that in mind, they would have realized the headlines carried less weight than in typical elections. Thus, the news reports were noisy, and opinion-makers should have seen that the election would be closer than the polls indicated.
The Good Judgment Project has arrived at that type of thinking with the help of scientists who came before them.
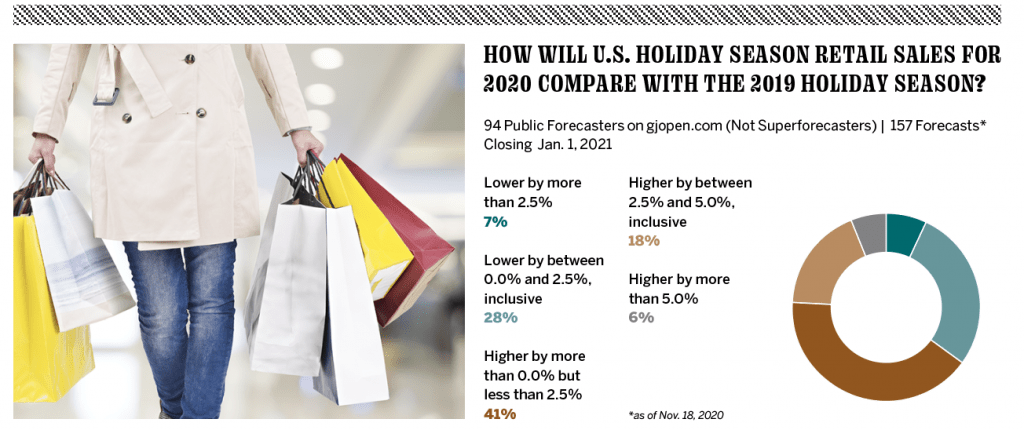
The project’s precursors
The origins of good forecasting stretch far back in time to methods like observing the flight patterns of birds to predict coming storms. It continued during the Renaissance with the development of calculus, the study of how things change.
Probability theory came along in the 17th century, and modern statistics with its standard deviations made its debut in the late 19th and early 20th centuries. Future studies came of age after WWII and gave rise to futurists like Alvin Toffler.
Predictive analytics and data-based forecasting came along in the late 20th and early 21st centuries and brought more-structured and quantifiable forecasts—the quantification of forecasting by assigning probabilities.
Giants in the progression of forecasting have included Isaiah Berlin, who published an essay called The Hedgehog and the Fox, which grew into a book by 1953. Its roots were in the ancient Greek idea that a fox knows many things, but a hedgehog knows one important thing. He divides writers and thinkers into two groups: those who view the world through the lens of a single idea and others who draw upon many experiences.
Noted forecaster Nate Silver advises in his book The Signal and the Noise that readers become “more foxy.” He even uses a fox as his logo on the fivethirtyeight.com site.
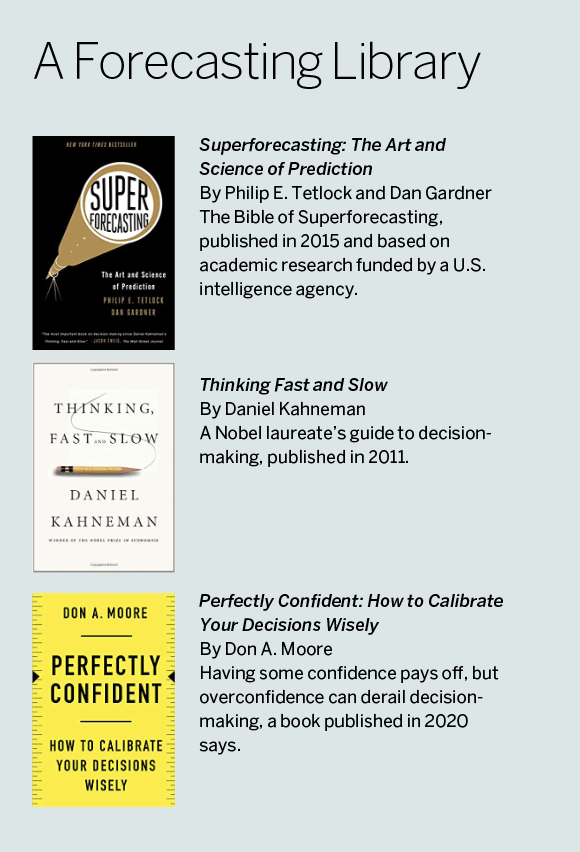
Daniel Kahneman, a scientist mentioned earlier, established a milestone in forecasting in 2011 with publication of the book Thinking, Fast and Slow. Kahneman, an Israeli psychologist and economist, had already shared the 2002 Nobel Prize in Economic Sciences with Vernon L. Smith. In Kahneman’s research into the psychology of judgment and decision-making, he often collaborated with Amos Tversky, another Israeli psychologist.
In Thinking, Fast and Slow, Kahneman sets forth the notion that people bounce between two modes of thought. First, there’s “fast,” which he viewed as instinctive and emotional. Second, there’s “slow,” a more deliberate and logical approach.
Elsewhere, Kahneman and Tversky explored cognitive biases, prospect theory and happiness.
Cognitive bias, a common condition, can lead to an irrational “subjective reality” that deviates from norms. Conversely, people sometimes manage to capitalize on cognitive bias, using it to save time, Kahneman and Tversky wrote in 1972.
The duo described prospect theory in 1979, and it became a factor in Kahneman’s 2002 Nobel Prize. It describes the behavior of people who are averse to risk-taking and also give too much weight to events with low probability and not enough to events with high probability.
Happiness became more than a vague state of mind for Kahneman, whose quantitative study of well-being, quality of life and satisfaction defined happiness.
Another scientist who contributed to the understanding of the clear thinking that’s needed for sound forecasting was Gary A. Klein, author of Sources of Power: How People Make Decisions. He established that what passes for intuition can actually be a decision based on years of experience and fact-gathering.
These scientists, as well as many others, contributed to the notion that good forecasting becomes a matter of distilling events to their essence.
The gist
Researchers that include Tetlock and Kahneman are working on what’s called “gisting,” the act of peeling layers from an issue to reach its core. If they can reduce a 1,000-word report to 10 words, they can filter out the noise and accelerate the sharing of information.
Applying the act of gisting to the work of Good Judgment Inc., one key player in the academic and business scenes summarizes the essence of the enterprise this way: “Forecasting is a skill that can be cultivated, and it’s worth cultivating.”
Forecasting as a Livelihood
Good Judgment Inc., a commercial enterprise that provides forecasts and forecasting training, bases its services on the knowledge, wisdom, expertise and personnel of the Good Judgment Project’s academic research team.
The company calls upon about 140 Superforecasters to predict events for dozens of industrial and governmental clients in North America, Europe and the Middle East, says CEO Warren Hatch. It also teaches the art and science of forecasting to clients’ staffs, he says.
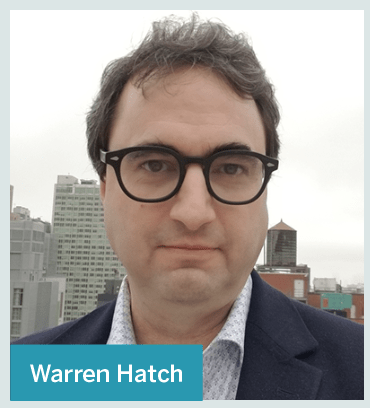
Hatch came to the helm through a natural progression. He began his academic career at the University of Utah and went on to earn a doctorate in politics at Oxford. Then he embarked upon a career as a Morgan Stanley analyst and portfolio manager.
But new challenges awaited. Hatch had read Expert Political Judgment, a book by Philip Tetlock, one of the founders of the Good Judgment Project academic team. Discovering that the project was seeking volunteers who liked to predict geopolitical events, Hatch signed up.
As a volunteer, Hatch compiled such an outstanding record of prediction accuracy that the project recognized him as among the top 2% of its participants and proclaimed him a Superforecaster.
When the academic project spun off the company, Hatch became one of the first full-time employees and has since risen to the top spot in the business. He now oversees Superforecasters who come from differing backgrounds and possess divergent habits of mind. But all share a love for pursuing and manipulating information. A third have bachelor’s degrees, a third have earned master’s degrees and a third have obtained one or more doctorates.
The company uses skilled generalists among the Superforecasters to bring “fresh eyes” to a subject. That means they’re not encumbered by preconceptions that can become “blinders” to viewing a problem or situation, Hatch observes.
“One of the major findings of this research is an increased awareness of this collective wisdom of the crowd and that subject-matter expertise is not necessarily a prerequisite to be able to add value to the forecasting process,” he notes. The only exception comes with highly technical issues—novices may need more time to understand the question than to forecast the result.
But the occasional technical roadblock doesn’t faze Hatch, who remains focused on employing the theoretical to interpret practical challenges. “How can you apply your findings in real-world situations to improve real-world decisions?” he asks rhetorically. “That’s been really exciting to do.”