The Future of Policing has Arrived
Predictive analytics warn of criminal activity from the streets to the boardroom
Computers are predicting when and where crimes will occur—but don’t expect a real-life remake of the 2002 sci-fi film Minority Report.
In other words, Tom Cruise won’t be swooping down in a futuristic bladeless helicopter to stop an aggrieved husband from plunging a pair of scissors into the chest of his cheating wife.
Instead, police are driving their cruisers through certain neighborhoods more often at a particular time on a specific day or night. And it works—statistics indicate the mere presence of law enforcement deters bad guys from doing wrong.
“We probably disrupted criminal activity eight to 10 times a week,” observed Sean Malinowski, formerly a captain in the Los Angeles Police Department Foothill Division. His testimonial to crime-prediction technology appears on the website of a company that supplies the software.
Such programming, often called predictive analytics, is demonstrably correct at predicting crime 90% of the time, according to University of Chicago researchers. Other experts contend it’s only a little better than guessing—but still significant.
They agree it’s important because reducing wrongdoing always pays off in this crime-ridden country.
Last year, homicide claimed the lives of 13,537 victims in the United States, according to reports filed with the FBI’s Crime Data Explorer website. But the law enforcement agencies providing the data cover only 64% of the nation’s population, so the real total would be much higher. Numbers derived from a full accounting would most likely surpass the 16,899 members of the American military who died in Vietnam in 1968. That was the conflict’s bloodiest year, according to the National Archives.
Plus, those stats don’t take into account the toll of lesser but still heinous violent crimes like manslaughter, forcible rape, robbery and aggravated assault. Then there are property crimes like auto theft, burglary and vandalism. And don’t forget the white-collar crimes of fraud, embezzlement, money laundering, securities and commodities scams, and theft of intellectual property rights.
Meanwhile, more crime has moved online during the pandemic in the form of identity theft, phishing, extortion, romance scams, confidence schemes, fake investments, and failure to pay for or deliver goods. In 2020, victims filed 791,790 complaints of suspected internet crime with the nation’s 18,000 law enforcement agencies, up from about 300,000 in 2019, according to the FBI. Losses in 2020 exceeded $4.2 billion, the bureau said.
So, what’s to be done?
Help is on the way
Geolitica, a 10-year-old Santa Cruz, California-based predictive policing company formerly called PredPol, is working with police departments and security companies to head off property crimes.
Nearly 50 police forces and sheriff’s departments are using the company’s service to help protect about 10 million citizens, said Geolitica CEO Brian MacDonald.
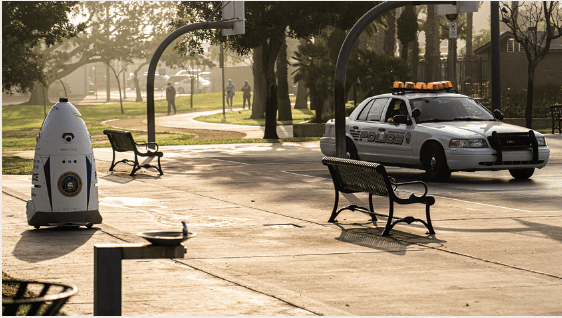
He declined to discuss how much his company charges but acknowledged that an initial cost of $60,000 posted on his website has increased of late.
Whatever the price, Geolitica gathers and crunches huge amounts of data to predict when and where crimes are most likely to occur.
“We start with two really basic concepts,” MacDonald said. “The first one is known informally as the law of crime concentration, which states that crimes do tend to cluster. Somewhere around 2% to 5% of a city generally generates more than half of any designated type of street crime.”
The law of clustering has been documented scientifically in the work of David Weisburd, a noted criminologist at George Mason University and Hebrew University in Israel.
Geographic hot spots include anything from obviously troubled neighborhoods plagued by socioeconomic woes to relatively clean and seemingly secure Walmarts where criminals break into shoppers’ cars, MacDonald noted.
“The second principle is that you can actually deter crimes by putting someone in those places before the crimes occur,” he continued. “Rolling a black and white through a parking lot with a lot of auto thefts on a random, irregular basis throughout a shift has proven to have a strong deterrent effect on crime.”
Christopher Koper, also a George Mason professor, conducted research in Milwaukee supporting this premise. If officers spend 10 to 15 minutes in an area, they have a chilling effect on crime for about two hours, Koper found. It’s what MacDonald calls “the center of the bell curve in terms of presence and deterrence.”
The two-step approach of identifying high-crime areas and then policing them prevents street crime but doesn’t deal with vice, like prostitution or drug peddling, and doesn’t prevent crimes, such as forgery, domestic violence or cybercrime, MacDonald noted.
What’s more, the data underlying the company’s work springs from police reports filed by citizens who are clearly victims. People almost always call the authorities to report a stolen car but seldom turn themselves in for smoking crystal meth.
The data set
Geolitica extracts three elements from police reports: the type of crime, the place where it occurred, and what day and time it went down, MacDonald said. Stated concisely, they’re focusing on “what,” “where” and “when” and leaving specific information about victims, perpetrators and neighborhoods untouched.
Crime clusters occur around the same time and in the same locations, he said, explaining robberies tend to occur downtown on weekdays around noon or in entertainment districts at night and on weekends.
In one example of typical criminal behavior, burglars who hit commercial enterprises instead of residences often move around a city, MacDonald noted. Veteran police officers know those patterns from experience and proactively work those areas at those times, even without the help of predictive analytics.
But relying on hunches opens the door to bias and thus makes officers less effective, he said. Plus, putting pins in a wall-mounted squad room map to locate high-crime areas is backward-looking and doesn’t point to days of the week or times of day.
Automating the process, as Geolitica does with predictive analytics, enables police departments or sheriff’s offices to draw upon much more data than people can compile and interpret on their own, he maintained.
But how does it work?
Crunching the data
When a law enforcement agency signs on with Geolitica, it provides two to five years of data. The company uses the stats to build a model that’s updated every day. If a department has three, eight-hour shifts on 10 beats, it receives 30 sets of predictions daily.
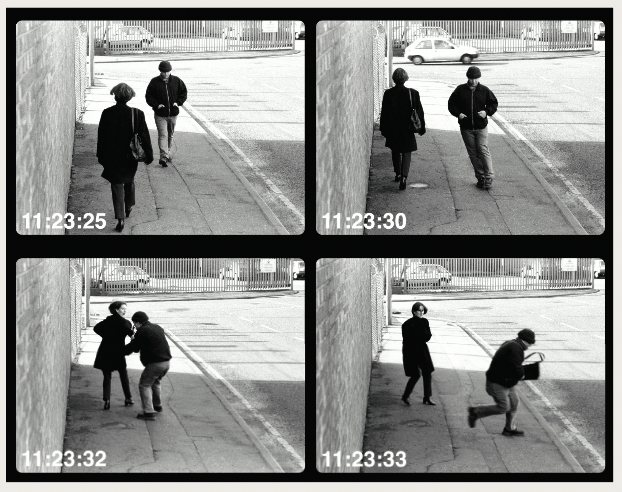
The predictions look like a Google map with two or three boxes, each encompassing a chunk of the city measuring 500 feet by 500 feet. The probability of specified crimes is greatest inside those boxes.
Between radio calls from the dispatchers, officers are expected to spend more time patrolling those areas and engaging with citizens there.
At the start of a shift, sergeants and patrol officers click on the boxes to see what crimes have high probabilities, said Deputy Police Chief Rick Armendariz of the Anaheim, California, police department. He implemented the Geolitica system while serving in the police department in Modesto, California.
During a six-month period, the Modesto department tracked auto theft, residential burglaries and commercial burglaries and managed to reduce each category by 40% to 60%, Armendariz said in an International Association of Chiefs of Police convention presentation. But the benefits didn’t end there.
“It made our department mission-specific,” he observed. Instead of allowing Modesto officers to create their own “missions” as self-appointed specialists pursuing crimes of their own choosing, like drug arrests or traffic stops, it improved the department’s efficiency by keeping the entire force “on the same page” and sending members out to follow the same priorities, he continued.
High-tech preventive policing seemed natural to Modesto’s younger officers but required a cultural shift for veterans of the force who were accustomed to relying on their personal knowledge of the city, Armendariz noted.
But even when officers want to follow the system’s mandate to spend time in certain areas, they’re often taken elsewhere in response to calls, noted Philip Lukens, chief of police in Alliance, Nebraska. Sometimes, they simply forget to follow up on the predictions, he said.
“If it’s up to the officer to look at the map and then go patrol that area, it just doesn’t happen,” Lukens maintained.
If an officer needs to work traffic from
3 p.m. to 3:15 p.m. at a certain intersection on a particular date, there’s an 80% chance of missing the appointed task, he said, adding that sergeants are simply too overburdened to make sure officers are complying.
That’s why Alliance is working with Geolitica to set up automatic “ghost calls” to dispatch an officer to the area at the right time. Instead of relying on officers to patrol a designated location, the system occasionally dispatches them to that spot. If the officer who would be receiving the call is otherwise engaged, the ghost call rolls to another officer.
“We need to pull and push data at the same time because that’s how we become effective at intercepting the issue,” Lukens said, “and then we’re analyzing it to see if we’re effective.”
His department also uses the preventive policing data to locate hot spots and then park an unstaffed, marked police car in the area as a deterrent to crime during a 6-hour to 8-hour window. The practice of using squad car decoys has reduced property crime in Alliance by 18% this year, he said.
But police may someday act upon preventive analytics without having to commit an officer or even an empty squad car. They could dispatch robots to do it.
A real-life R2D2
Knightscope, a Mountain View, California-based public company, began operations in 2013 and deployed its first crime-preventing robots two years later, according to CEO William Santana Li.
“Our mission is to make the U.S. the safest country in the world,” Li said. “What if a robot could save your life?”
The company’s robots operate on predictive policing data from other companies to make their rounds. They come in both indoor and outdoor models, and the most popular, known as the K5, stands 5 feet tall, measures 3 feet wide, weighs in at 400 pounds and roams around autonomously.
The robots combine four difficult-to-execute technologies: robotics, electric vehicles, artificial intelligence and autonomous self-driving capability, Li said.
Knightscope doesn’t sell the robots but instead rents them out for 75 cents to $9 an hour, depending upon the application. The company provides the hardware, software, docking stations, telecom connections, data storage, support and maintenance.
About 100 of the roving robots are on the job, joining the company’s thousands of stationary devices that are capable of such tasks as facial recognition and capturing license plate numbers.
Some police departments are using the mobile robots to patrol areas where predictive analytics forecasts crime is most likely to happen, Li said, offering anecdotes about the machines assisting in the arrest of a sexual predator and an armed robber.
A unit nicknamed “HP RoboCop” has made the rounds at a city park in Huntington Park, California, urging in a pleasant voice that people “please help keep the park clean.” The city is spending less than $70,000 a year to rent the device, Cosme Lozano, the town’s chief of police, told a local NBC News outlet before the pandemic.
Vandals once tipped over HP RoboCop, but the machine recorded images of its attackers on videotape, and the police took the alleged miscreants into custody within hours of the incident, Lozano said.
Effective police work aside, most Knightscope units are finding homes in casinos, hospitals, warehouses, schools and apartment complexes—relieving guards of the monotonous duty of monitoring large spaces.
Li estimates 90% of the robots are working in the private sector and 10% for government agencies, including police departments. But he envisions having a million of them on the job in the next two decades, aiding law enforcement officers and security guards.
Whether robots proliferate or not, using predictive analytics to deter wrongdoing doesn’t end with heading off street crime. Banks can use it to detect and prevent money laundering, and insurance companies can use it to set rates keyed to the likelihood certain customers will commit fraud or take up dangerous lifestyles.
White-collar crime
Businesses are using computing power to enhance their ability to deal with malfeasance, according to Runhuan Feng, a University of Illinois mathematics professor. He directs the school’s new master’s degree program in predictive analytics and risk management for insurance and finance.
For many years, bankers and insurers have relied on imprecise rules-based pattern recognition in their attempts to curb impropriety. They might, for example, flag an account when a depositor transfers more than $10,000, Feng said.
But unaided humans can’t apply enough rules to achieve much accuracy. Now, however, computers are amassing and evaluating enough data to create models that generate much more valid predictions.
Such predictions are benefitting an insurer that sought Feng’s advice. He not only helps the insurance company manage its own data but also adds data from other sources. That results in better models that identify riskier customers who should pay higher rates. It alleviates the need for lower-risk customers to subsidize those more likely to indulge in fraud.
Fraud constitutes a real problem for insurers because criminals file false claims with several companies, he noted.
Yet, fraud isn’t the only reason insurance claims can increase in number or severity. In one example, bringing in information from third-party sources might reveal that a customer engages in extreme sports, Feng said. That means the insurer should charge a higher premium for life or health coverage, he noted.
Before the insurance company approached Feng, it was predicting fraud and other problems with 50% to 60% accuracy, not much better than flipping a coin. The new models get it right more than 90% of the time, Feng said.
Meanwhile, better models can also alert banks to money laundering.
“What usually happens is these individuals get money from illegal activities,” Feng noted, “and they open lots of accounts and deposit small amounts. Then they use them to buy luxury products and sell them quickly.”
The machine learning model captures and combines the extensive and far-flung information needed to establish that sort of pattern and link it to an individual and compare it to known money laundering, he observed.
Few would argue against halting money laundering activities or preventing fraud, but some still aren’t comfortable with using predictive analytics to foil criminal activity. Critics view it as an authoritarian “Big Brother” approach to invasive surveillance.
Multiple sources note that discrimination can come into play with predictive analytics.
But proponents have responses to those concerns.
Bias and invasion of privacy
Racial bias can creep into predictive policing. Including drug arrests in the data set, for example, introduces bias against African Americans because they’re more likely than members of other ethnic or racial groups to be charged with possession or selling, according to many skeptics.
“It has nothing to do with whether there are more drugs in those neighborhoods,” MacDonald said, “it just happened to be where those officers made more arrests for that particular crime.”
That’s why Geolitica doesn’t include arrests in its data gathering, instead relying solely on complaints phoned in to police dispatchers, MacDonald said. Besides, police often arrest suspects far from where the crime occurred and almost always after the fact, not during the commission of the crime.
But fear of bias has prompted some municipalities to ban predictive policing. Santa Cruz, California, home of Geolitica, began testing the technology in 2011, suspended its use in 2017 and banned it
by city ordinance in 2020, according to the Los Angeles Times.
The technology became particularly invasive in Chicago, where authorities went so far as to notify individuals that they were likely to become involved in a shooting. The authorities just didn’t know from the predictive analytics which side of the gun the citizen would be on. By some accounts, the list included more than half of the
African American residents of the city, said multiple sources.
“If you have this kind of predictive analytics, then does that give the state too much power?” asked Ishanu Chattopadhyay, a University of Chicago professor who’s spent the better part of a decade researching the subject. “Are the cops going to round people up? Put them in jail? Are we going to be in a Minority Report world?”
Chattopadhyay’s research team came under fire from skeptics this summer when it published the results of its predictive policing research in Chicago and other cities. He reported receiving “hate mail written in very polished language.”
The letter writers had not read the team’s report, he speculated, or else they would have realized that the research centered on aggregated statistics instead tracking individuals.
Still, Chattopadhyay takes exception to identifying crime hotspots. They become a self-fulfilling prophecy because if you’re looking for crime more often in an area, you’re likely to find it, he said.
At any rate, he explained the results of his research by saying that if 10 crimes are going to happen in the future, his team flagged 11 crimes and was correct eight times, had mixed results three times and false positives twice. Those results were presented in media reports as being 90% accurate.
The predictions, which produced a flurry of news coverage a few months ago, were made seven days in advance, plus or minus one day, and they were restricted to a couple of city blocks.
So complaints aside, the tools for predictive policing have become a reality that’s unlikely to disappear, according to Chattopadhyay.
“Some people are upset about AI in general and see it taking over their lives,” he said, “but artificial intelligence is here to stay. The real question is, are we going to go for the worst possible outcome or make the best of it?”
Predictive Analytics: When Machines Go to School
Crunching numbers to predict the future isn’t limited to forecasting crime. It can tell us, for example, that vegetarians miss their flights less often than meat eaters. (True, by the way.)
Who would care about that? Well, that type of granular information helps airlines set ticket prices and adjust schedules, thanks to something called “predictive analytics.”
It’s a phrase that might seem vague, so Luckbox turned to an expert to pin down the definition. He’s Eric Siegel, a former professor at Columbia University and author of Predictive Analytics: The Power to Predict Who Will Click, Buy, Lie, or Die.
“Predictive analytics is essentially a synonym for machine learning,” Siegel said. “It’s a major subset of machine learning applications in business.”
Machine learning occurs when computers compile data, and data is defined as a recording of things that have happened, he continued. It renders predictive scores for each individual—indicating how likely customers are to miss a flight, cancel a subscription, commit fraud or turn out to be a poor credit risk.
“These are all outcomes or behavior that would be valuable for an organization to predict,” Siegel noted. “It’s actionable because it’s about putting probabilities or odds of things.”
Plus, predictive analytics isn’t just about humans. It can forecast the likelihood of a satellite running out of battery power or a truck breaking down on the highway.
Just about every large company uses it, and most medium-sized ones do, too. Cell phone providers predict which customers will switch to another network, and FICO screens two-thirds of the world’s credit card transactions to understand who might commit fraud.
“Prediction is the holy grail for improving all the large operations we do,” Siegel maintained. “It doesn’t necessarily let you do it in an ‘accurate’ way in the conventional sense of the word, but it does it better than guessing.”