When Models Fail
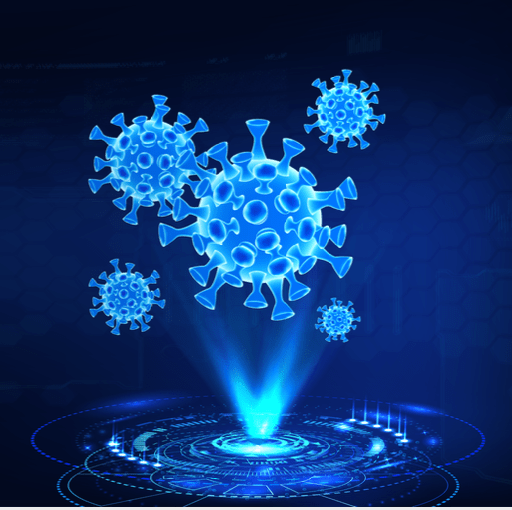
Models aren’t just for pricing options—they can (sometimes) predict the spread and mortality of a pandemic. Just keep expectations in check. Models, by definition, are not precise and, inevitably, the people who rely on them seem less than perfect.
Here’s a fact: The people who attack the models predicting the number of infections and deaths from COVID-19 are just plain wrong. I’m not a virologist, immunologist or epidemiologist. But I do build models—financial models that hundreds of thousands of traders rely on to find profits.
I understand and take full responsibility for what the models I create can and cannot do. And while I’m not in the medical field, I do understand the core mathematical model of viral or bacterial growth, and why attacking it makes no sense.
The basic argument against the models goes something like this: In early March 2020, the mathematical epidemiologist Neil Ferguson and his team at the Imperial College of London published a report stating that COVID-19 could kill more than 500,000 in the U.K. and more than two million in the United States.
That prompted governments big and small to “close” the economy, causing the market to crash and putting millions out of work. The actual number of deaths from COVID-19 is a tiny fraction of those projections, causing many to complain that the model was wrong and that the misery from the closed economy falls on the head of the scientists. They should be pilloried—both figuratively and literally, those critics insist.
Ugh. Excuse me while I pound my head on my old calculus book.
How models work
Just like option pricing models used by traders—like Black Scholes and binomial—several models predict the spread of a virus in a population, such as SIR and logistic. But just like those option pricing models, the virus models have the same core, which is an exponential function.
To understand an exponential function, ex, think of a line that starts low and then curves upward, steeper and steeper the farther it goes. Exponents are everywhere. A bank account grows exponentially. Stomp on the gas, and a car accelerates exponentially.
They’re very reliable tools for modeling all sorts of phenomena. Sure, the models used by the epidemiologists are more complex. But exponential growth means one sick person can turn into a thousand very quickly.
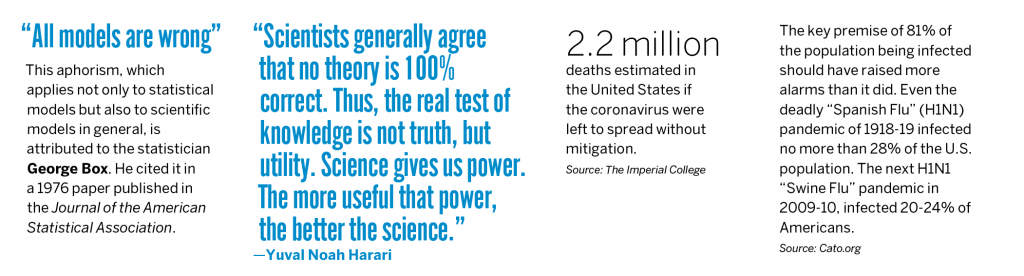
For example, using a simple exponential function: e where is the rate of the daily spread of the virus and is the number of days in the future, see what happens when one sets to 60 and to .02 and then .05. e.02*60 = 3.32. e.05*60 = 20.08.
Those numbers mean that if 100 people are infected now and the spread grows by 0.02, then 332 people will have the virus in 60 days. With 100 people infected now and the spread growing by 0.05, 2,008 people will have the virus in 60 days.
In an exponential model, a small change in growth creates big changes in the results. Apply those numbers to the 350 million people in the United States, and it’s easy to see why two million fatalities wasn’t a long reach.
Epidemiologists try to figure out . That’s where the complexity sets in. They estimate the probability of one person spreading the virus to another and the number of people each person might come into contact with. They do that by looking at the data of number of sick versus healthy people, and the number of sick people who die versus those who recover.
In the real world
With the data that was available in February and early March, the Imperial College of London gave its best estimate of what the coronavirus might do. Later, as new data came in and was incorporated into the model, the model came up with a different, smaller number of potential fatalities.
Critics attacked this as “walking back” the original estimate as though it was wrong and that the ICL should be embarrassed. Far from it. When new data is available, it’s input into the model for a new estimate. That works in epidemiology, science and finance.
Don’t blame the COVID-19 models. The problem is with a government and media that fail to put the models’ results into context.
New data improves the model
Imagine a hurricane developing far out in the Gulf of Mexico. It might strike Mississippi, Louisiana or Texas. As it moves closer and data is collected, its predicted landfall gets narrower and narrower. Crude oil refineries on the coast rely on those models. Coronavirus models work the same way.
Models, then, provide a guide. They’re meant to be predictive and helpful in making decisions. Models aren’t wrong unless the math underlying them is wrong.
Some models do indeed fail. But they tend to fail quickly and disappear from the arena for which they were created.
The models for the spread of a virus, on the other hand, have been used successfully to model all sorts of outbreaks. And they get better each time. So, it makes sense to use them for the coronavirus.
Blaming the model
Critics commingle political frustration with the model. The model isn’t the problem. Neither is the scientist. The problem is with a government and with media who don’t know how to put the model’s results into context.
A model doesn’t say something will happen—only that something might happen if things go the way the inputs suggest. Would people prefer the answer “some” to the question “How many people might die from COVID-19?” Of course not!
Models in any framework quantify various outcomes as either likely or unlikely. It’s up to people to use that information to judge whether the benefit of a course of action is worth the risk.
In trading, just because some SPX put has a 95% probability of expiring worthless doesn’t mean investors take all of their capital and use it to short as many of those puts as possible. There’s more to it.
Why? Because sometimes the SPX has one of those huge drops that happen only 5% of the time, and that could put a trader out of business. So, they put that 95% into context and perhaps sell one of those puts using a small percentage of their capital.
That’s called risk management, and it’s a smart way to use probabilities. Yes, trust the models that generate those probabilities, but understand that they’re not perfect.
So, anyone who doesn’t like the way the response to COVID-19 has been going can take it out on the politicians in November—not on scientists and their models.
The Mathmatics of Coronavirus
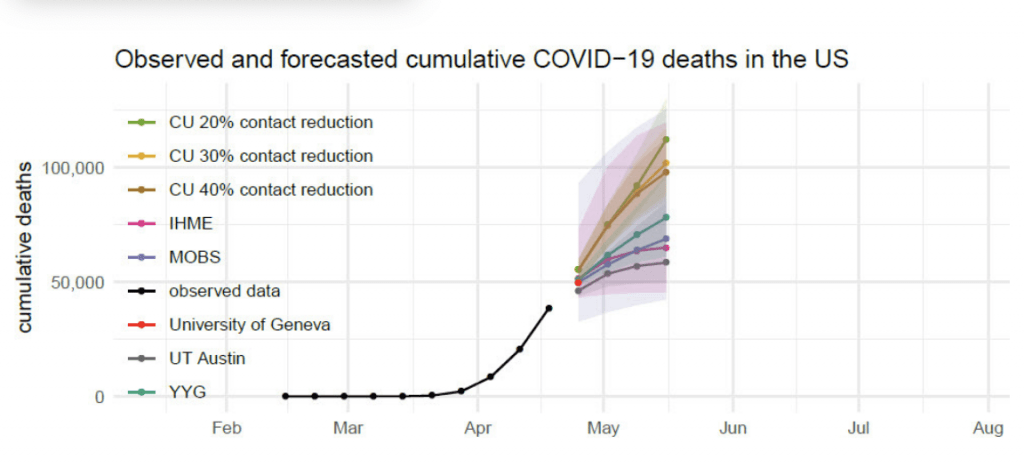
During a pandemic, real data becomes hard to find. Chinese researchers have published only some of their findings on the spread of COVID-19 in Hubei. The ongoing catastrophe of testing for the virus in the United States means no researcher has a reliable denominator, an overall number of infections that would be a reasonable starting point for untangling how rapidly the disease spreads. Since the 2009 outbreak of H1N1 influenza, researchers worldwide have increasingly relied on mathematical models, computer simulations informed by what little data they can find, and some reasoned inferences. Federal agencies like the Centers for Disease Control and Prevention and the National Institutes of Health have modeling teams, as do many universities.
—Wired.com
Tom Preston, Luckbox contributing editor, is the purveyor of all things probability-based and the poster boy for a standard normal deviate. tp@tastytrade.com