Data Mining Diversity. Caution: May Contain Lies.
Either through luck or subterfuge, stats can “prove” a thesis that’s simply not true as illustrated in this behind-the-scenes look at how a retail investment fund was inappropriately marketed to unsuspecting investors. The lesson? Data is not evidence: It may be the result of data mining.
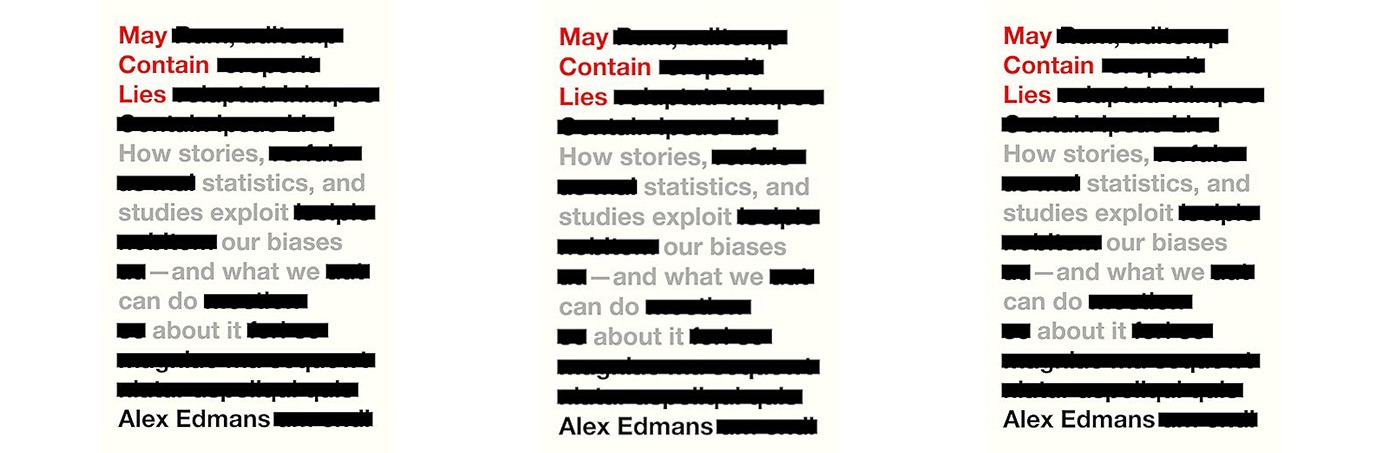
We mentioned in last week’s Luckbox we were reading May Contain Lies by Alex Edmans with the intention of reviewing the book in an upcoming issue. (Spoiler Alert: It’s going to be a very good review.) To entice readers to pick up your own copy, we reached out to the author and he graciously granted us permission to share this excerpt with you.
***
Few opportunities in life are truly life-changing, but my coffee appointment at the Dorchester Hotel on London’s Park Lane had the potential to be. A few weeks earlier, when cleaning up my junk-email folder, a message caught my eye. Among the notifications of a lavish inheritance, pleas for life-saving donations and promises of unconditional love, one email stood out because of the sender’s name: It was from one of the most famous investors in the world. I braced myself for an investment scam launched under a false identity. Otherwise, what would a powerful money manager want from a lowly assistant professor? But when I opened the email and read its contents, it seemed genuine. We exchanged messages, which gave me further confidence, and when the sender asked to meet in person, I was thrilled.
This was a meeting I didn’t dare be late for, and I arrived nearly half an hour early. I waited in the plush, bright lobby of the £800-a-night hotel where the investor was staying, gazing at the high ceilings and looking through the front window toward the green outline of Hyde Park in the distance. Our meeting time of 10 a.m. came and went, and the doubts started to creep in. The emails appeared authentic, and the sender seemed to know my research. Perhaps this was naïve acceptance on my part—I wanted the messages to be genuine. My rational System 2 kicked in for the first time since receiving that email, and I wondered if I’d been duped.
Ten minutes later, the investor arrived, looking the very image of a master of the universe—radiating both authority and calm. The financier, whom I’ll call Xinyi, wished to launch a fund that backed pro-diversity companies, and wanted evidence to buttress her strategy. She’d heard of my research showing how the 100 Best Companies to Work for in America—firms that go above and beyond in how they treat their employees—beat their peers, and hoped this might be what she needed. I explained that the Best Company assessment does take diversity into account. Yet it’s far more than that, and it’s impossible to know whether it was diversity, or the other aspects of being a Best Company, that drove the outperformance I’d found. Employee satisfaction is granular, and diversity is only one item under the umbrella.
Undeterred, Xinyi asked if I could adapt my methodology to conduct a new study, focused specifically on diversity. I said I could, by replacing Best Company status with a diversity measure, and gave examples of the many ones available. Xinyi was enthused, and asked if I could perform the analysis. If it worked out, I could partner with her in the launch of this new fund.
I stepped out of that lobby walking on air. This was a golden opportunity, and if the results panned out, the benefits would be endless. I’d land a top publication, which would be highly cited, since diversity was a hot topic. Beyond academia, I’d work with one of the leading investors on the globe, who’d catapult me out of the ivory tower on to the main stage. I could be heralded as a champion for diversity, in turn opening many other doors. Quite apart from the instrumental pay-offs, diversity was something I was intrinsically passionate about. I’d always been one of the few non-white faces at school, at university, in investment banking, and even in my leisure time—such as on the football terraces where I had a season ticket in the late 1990s.
Given the many diversity measures available and thus analyses to run, I approached one of my Wharton MBA students to work with me, whom I’ll call Dave—because that’s his name. He had strong quantitative skills, having scored an A+ in my class, and a passion for ethical investing. We studied a Thomson Reuters database, which provides data across eighteen different dimensions of corporate responsibility, one of which was diversity. Within diversity, there were dozens of areas. Xinyi was particularly interested in gender, and we found 24 relevant measures. We crunched the numbers 24 times, hoping to strike gold.
But instead we struck mud. Out of those 24 measures, 22 were negatively associated with company performance.‡ For some of those 22, such as the percentage of female board directors, the relationships were statistically insignificant—sufficiently weak that they could have been due to chance. For others, like having a maternity-leave policy, the link was both negative and significant. Out of the two bright spots, the percentage of women managers had a positive link, but it wasn’t significant. However, there was one association that was significant in the direction we wanted—the number of diversity controversies reported in the media. The fewer the headlines, the stronger the performance.
It was clear what we should do if we wanted to work with Xinyi—report only the one positive and significant result. Or we might disclose both positive results, to give the impression of honesty, as we could concede that one was insignificant but we were reporting it for transparency. But my job as a professor was to do scientific research. Even if I only used the study to launch a fund and gave up on the idea of publishing an academic paper, I’d still be spreading misinformation. Research is research, regardless of what it’s used for, and scientists can’t just pick and choose the results they want.
We emailed all 24 results to Xinyi, who was disappointed but graciously thanked us for our efforts. Dave wrote up the results into a thesis for his MBA; I went back to my other projects, thinking this was the last I’d hear of it. Despite my hopes after the initial meeting with Xinyi, I took this failure on the chin. Over my short career, I’d already tested several hypotheses that didn’t work out, so I knew that disappointment was simply part of the research process.
Six months later, a news article grabbed my attention. Xinyi was launching a fund based on the premise that female-friendly companies perform better—the exact thesis our analysis had contradicted. She quoted research from a company I’ll call Fixit that claimed to find a huge effect of diversity on performance. Fixit used a diversity metric that was none of the 24 that Dave and I studied, and entirely different performance measures. Not surprisingly, Xinyi made no mention of the tests that Dave and I ran for her and which didn’t pan out.
This episode highlights a key reason why data is not evidence: It may be the result of data mining. Data mining is where researchers engage in a biased search for a particular conclusion—they conduct hundreds of different tests, hide those that don’t work and jump on the one that hits the bullseye. As a result, there’s a simple path to launching an influential paper—mine the data, hope and pray you’ll find something significant and report only that result.
In fact, you don’t even need to hope and pray. You just need to run enough tests. Even if there’s no true link between the input and output, one might arise in the data due to luck. If you toss a fair coin enough times, there will be streaks of six heads; if you test a hypothesis 100 ways, five of them will be significant at the 5% level, even if the hypothesis is false.* This means, on average, you only need to try 20 times to get what you want.† “If at first you don’t succeed, try, try again” isn’t just an abstract proverb—it’s true in real life when it comes to data mining.
How can you get enough tries to ensure you succeed? By experimenting with different measures. Both Xinyi and Fixit are guilty of data mining in this example—Xinyi hand-picked the Fixit research because it claimed what she wanted, and Fixit likely knew their study would be more impactful if it found significant results—but we’ll refer to Fixit, as they act ally crunched the numbers. Starting with the input, Fixit could have studied any one of the 24 diversity metrics in Thomson Reuters, plus the dozens, potentially hundreds, in other data- bases. They stumbled on one that gave them what they wanted—comparing companies with three or more female directors against those with zero.
Fixit also played around with the output: financial performance. There’s one indicator that’s head and shoulders above the rest, shareholder returns. That’s how much shareholders get from investing in the company, and so it was the only yardstick Dave and I studied. But Fixit used the profit margin instead.* The profit margin misses out dozens of other drivers of shareholder returns, such as future prospects, new product launches and management changes. Yet Fixit chose the profit margin because it worked. It was the one output that, by chance, happened to be correlated with their measure of diversity. Fixit’s results gave Xinyi what she needed to launch her fund, attracting millions from eager investors who lapped up her claims.
***
* Recall that for a link to be statistically significant, the likelihood it arose from pure chance must be 5% or less. Thus, there’s a 5% likelihood that one test yields a significant result due to luck. If you run a hundred independent tests, on average 5% × 100 = 5 will be significant.
† Out of 20 tests, on average one will uncover a significant link in either the positive or negative direction. If you want a positive and significant result, on average you’ll need to run 40 tests.
‡ Note this is a quite different problem to what we saw in Chapter 3, where studies used measures that had little to do with what they claimed. Here, all 24 were valid ways to gauge diversity, but we had the freedom to pick and choose the ones that worked.
Reprinted with permission from May Contain Lies: How Stories, Statistics, and Studies Exploit Our Biases—And What We Can Do about It by Alex Edmans, courtesy of the University of California Press. Copyright 2024.